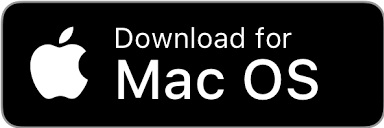
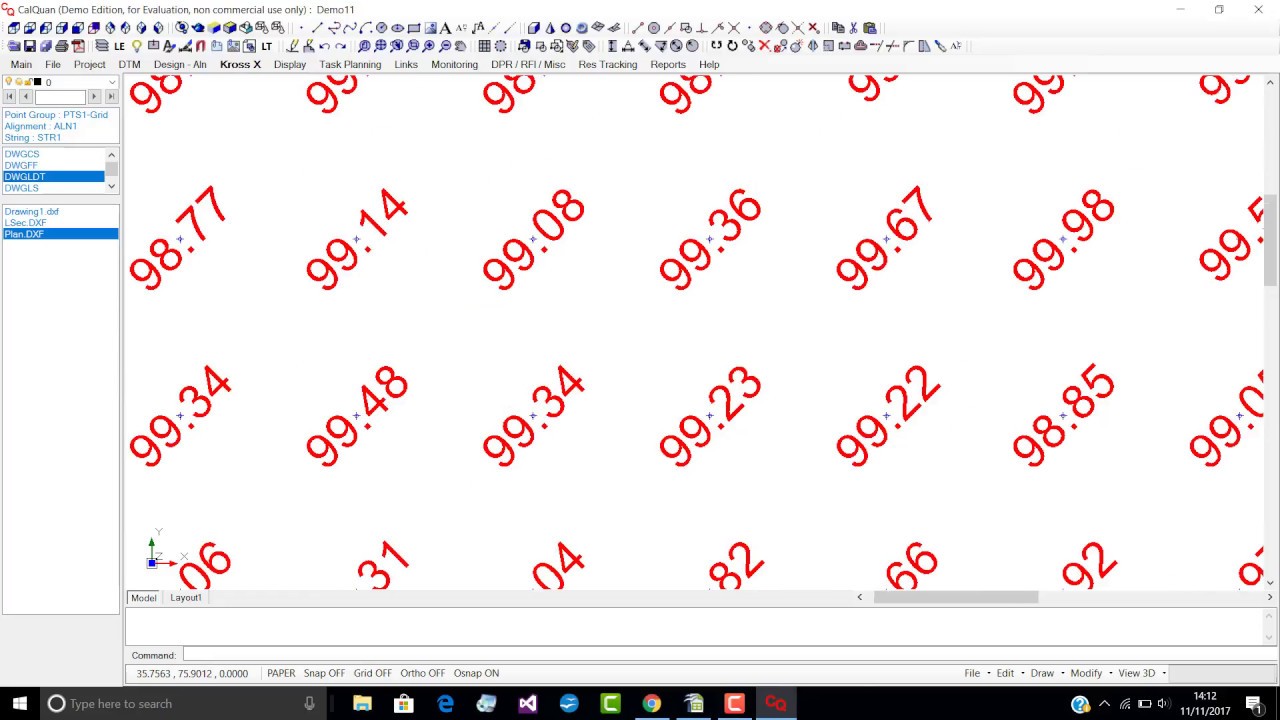
- Dim3 grid calculation how to#
- Dim3 grid calculation generator#
- Dim3 grid calculation serial#
- Dim3 grid calculation update#
- Dim3 grid calculation code#
My issue is that in this simple example I either get the created zero matrix as a result in Python or, if after the kernel call cudaDeviceSynchronize() is added, an illegal memory access error (Code 700).
Dim3 grid calculation code#
From this information, the process can compute the number of samples that it should check.Following the C++/CUDA extension tutorial on the pytorch website and having a look at the linked source code I have created my own CUDA kernel which does not do something useful, but is done as a learning project. Each process is assigned a unique rank and also knows the total number of processes.
Dim3 grid calculation how to#
It only gives us the means to accomplish inter-process communication, but it is up to us to decide how to distribute the work load. MPI, by itself, does not perform any parallelization of the code. This communication happens primarily over the network, but MPI also supports multiple processes on the same physical computer. The Message Passing Interface (MPI) is a library that allows different processes to communicate with each other. The way to get around this is to split up the computation among multiple physical computers.

The main downside of multithreading is that we are limited to the number of the relatively few computational cores available on a single CPU. This is because even though concurrent_threads returns 12 on my system, the CPU contains only 6 physical hardware cores. > a b plot(a,b/(b*a),type="o",xlab="num threads",ylab="efficiency", col="red")Īs can be seen from Figure 4, parallel efficiency significantly decreases with more than 6 cores.
Dim3 grid calculation generator#
This is because even though multiple threads are running, they are spending most of their time waiting for the generator to become available, instead of crunching through the samples.Ĭompiling and running the code, we obtain Without using this array, you would find that there is no speed up with more threads, despite the system monitor showing 100% utilization of all cores. This implies that only a single thread can access the generator at a time. After each value is sampled, the sequence index needs to be incremented. It is basically a function that samples consecutive values from a very large sequence. The utilized Mersenne Twister random number generator from the library is actually a pseudo-random number generator. One other change you may have noticed is that we are using an array of random number geneators, vector rnd. This will typically be twice the number of actual hardware cores. This default value represents the number of logical cores the CPU supports. The number of threads is obtained from hardware_concurency() function but can be overridden by a command line argument.
Dim3 grid calculation update#
Since the total desired number may not be evenly divisible by the number of threads, we update the count on the last thread to make sure we get the correct total. Also, each worker is given the number of samples to check. The main function then sums up the values in N_in to get the total. The reason for storing the threads in a vector is so that we can subsequently call join() to wait for the worker code to finish. Alternatively, we could use a class with an overloaded operator() but that leads to a slightly more complex code. For simplicity, here we use a regular function, and use an array of N_in to store the computed results. These (optional) arguments can also be passed in to the thread constructor. The constructor for std::thread requires any function-like (functor) object that can be called as Object(arg0, arg1, arg2. We launch this function in parallel by creating a new object of type thread.
Dim3 grid calculation serial#
The main difference from the serial version is that the computation has been moved to a Worker function. Schematic for computing π from area ratios Table of ContentsĪ simple way to estimate the value of π is from the area ratio between a unit circle and the enclosing unit square (or in this case, a unit quarter circle). The goal of that course is to provide students with the necessary computational background needed to tackle real computational projects. This topic is somewhat in line with the ASTE-499 Applied Scientific Computing Course I am currently teaching at USC’s Astronautical Engineering Department. This year, for “pi day” (March 14th), I figured I’ll post a short article demonstrating how to estimate the value of π using different computer architectures.
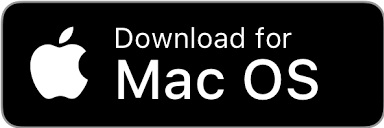